News Overview
Index 〉News overview page 〉Show item
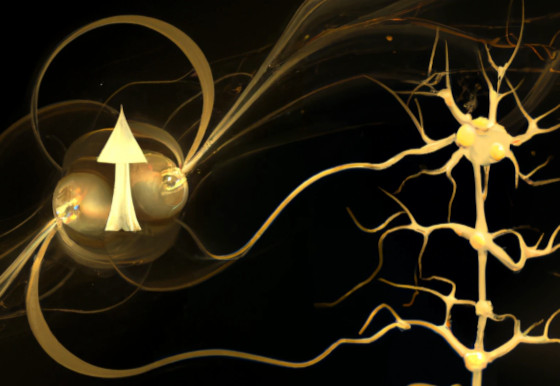
Summary by Tobias Schmale, Moritz Reh and Martin Gärttner:
Quantum simulators solve quantum many-body problems that are hard to simulate on classical computers due to the exponential increase of computational cost with the number of simulated particles. This exponential hardness also hits when performing quantum tomography, which means fully characterizing the prepared quantum states. Machine learning inspired variational approaches may overcome this challenge by restricting the manifold of trial states among which the experimentally prepared state is searched. The trick is to find structures inherent to the physical problem the simulator tries to solve, and to use these to create a compressed state representation, just like e.g. in image compression methods. In [1] we demonstrated that this method leads to a scalable tomography scheme using convolutional neural networks. We considered various experimentally relevant scenarios where we generated synthetic measurement data numerically. Working towards deploying this method on real experimental data, we recently applied it to an experiment creating entangled photon pairs [2]. A unique extension, that was possible thanks to collaborations within STRUCTURESis the use of neuromorphic hardware. Optimizing the parameters of an analog neuromorphic chip we demonstrated the encoding of Bell states [3] and quantum ground states [4].
Associated Literature:
- T. Schmale, M. Reh, M. Gärttner. Efficient quantum state tomography with convolutional neural networks. NPJ Quantum Information 8, 115 (2022).
- M. Neugebauer, L. Fischer, A. Jäger, S. Czischek, S. Jochim, M. Weidemüller, M. Gärttner. Neural-network quantum state tomography in a two-qubit experiment. Phys. Rev. A 102, 042604 (2020).
- S. Czischek, A. Baumbach, S. Billaudelle, B. Cramer, L. Kades, J. M. Pawlowski, M. K. Oberthaler, J. Schemmel, M. A. Petrovici, T. Gasenzer, M. Gärttner. Spiking neuromorphic chip learns entangled quantum states. SciPost Phys. 12, 39 (2022).
- R. Klassert, A. Baumbach, M. A. Petrovici, M. Gärttner. Variational learning of quantum ground states on spiking neuromorphic hardware. iScience 25(8), 104707 (2022).